Smap/sentinel-1: a two-layer machine learning-based framework with spatial/temporal transfer learning
semanticscholar(2019)
摘要
As the most recent 3 km soil moisture product from the Soil Moisture Active Passive (SMAP) mission, the SMAP/Sentinel-1 L2_SM_SP product has a unique capability to provide global-scale 3 km soil moisture estimates through the fusion of radar and radiometer microwave observations. The spatial and temporal availability of this high-resolution soil moisture product depends on concurrent radar and radiometer observations which is significantly restricted by the narrow swath and low revisit schedule of the Sentinel-1 radars. To address this issue, this paper presents a novel two-layer machine learning-based framework where the brightness temperature and subsequently the soil moisture are predicted at gap areas. Combined with the transfer learning strategy, the proposed method is able to gap-fill soil moisture with higher accuracy at areas where the radiometer observations are available while the radar observations are missing. The effectiveness of the two-layer framework is validated against hold-out SMAP/Sentinel-1 3 km soil moisture estimates at three study areas with distinct climate regimes, Arizona, South Dakota, and Arkansas. Results indicate that our proposed method is able to reconstruct 3 km soil moisture at gap areas with higher Pearson correlation coefficient (mean R = 0.723/0.82/0.798, at Arizona/South Dakota/Arkansas) and lower unbiased Root Mean Square Error (mean ubRMSE = 0.029/0.029/0.08) when compared to 1) the SMAP 9 km soil moisture product (mean R = 0.471/0.765/0.523, mean ubRMSE = 0.036/0.032/0.109) and 2) the conventional one-layer machine learning model that directly downscales soil moisture from 9 km to 3 km (mean R = 0.532/0.76/0.69, mean ubRMSE = 0.036/0.033/0.092).
更多查看译文
AI 理解论文
溯源树
样例
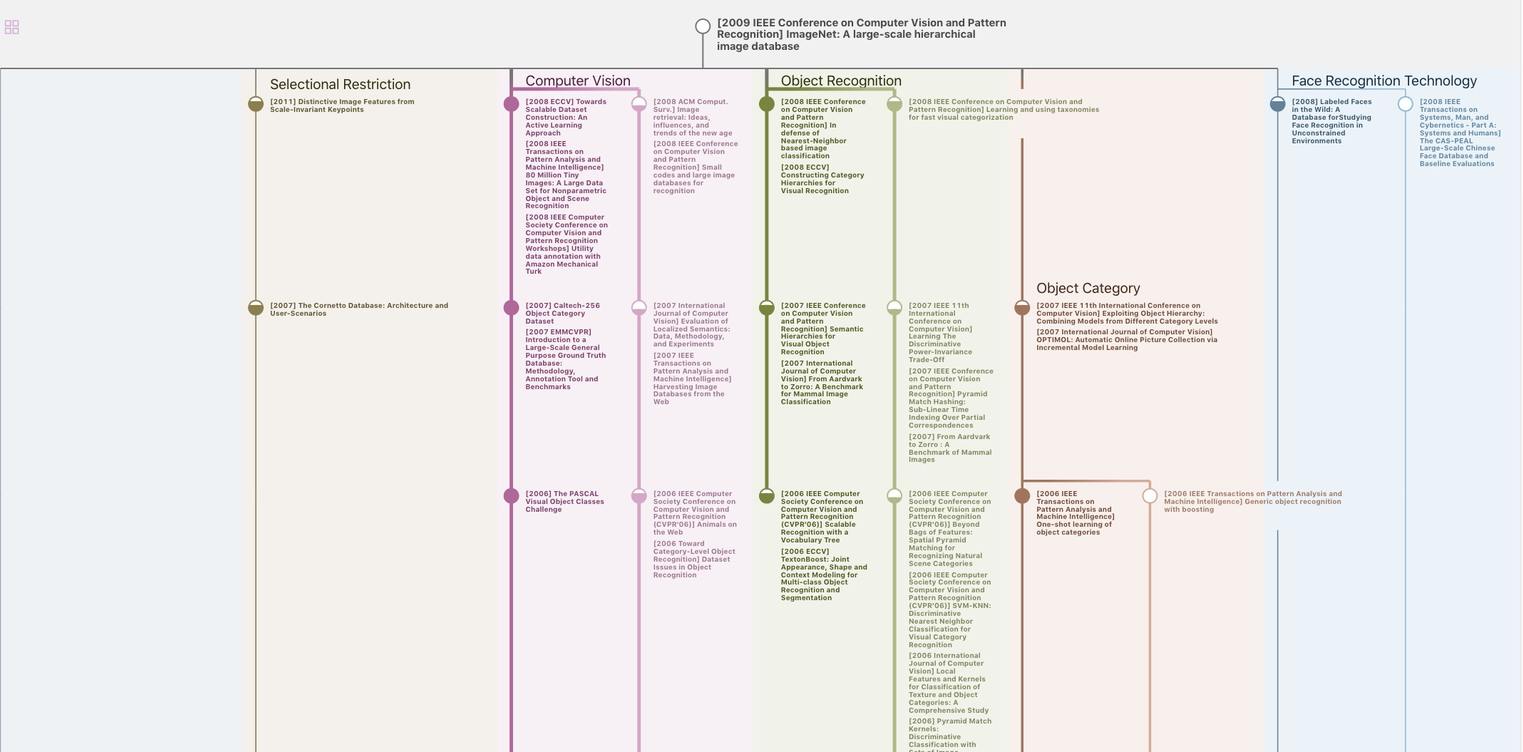
生成溯源树,研究论文发展脉络
Chat Paper
正在生成论文摘要