A survey on multi-objective hyperparameter optimization algorithms for machine learning
Artificial Intelligence Review(2022)
摘要
Hyperparameter optimization (HPO) is a necessary step to ensure the best possible performance of Machine Learning (ML) algorithms. Several methods have been developed to perform HPO; most of these are focused on optimizing one performance measure (usually an error-based measure), and the literature on such single-objective HPO problems is vast. Recently, though, algorithms have appeared that focus on optimizing multiple conflicting objectives simultaneously. This article presents a systematic survey of the literature published between 2014 and 2020 on multi-objective HPO algorithms, distinguishing between metaheuristic-based algorithms, metamodel-based algorithms and approaches using a mixture of both. We also discuss the quality metrics used to compare multi-objective HPO procedures and present future research directions.
更多查看译文
关键词
Hyperparameter optimization, Multi-objective optimization, Metamodel, Meta-heuristic, Machine learning
AI 理解论文
溯源树
样例
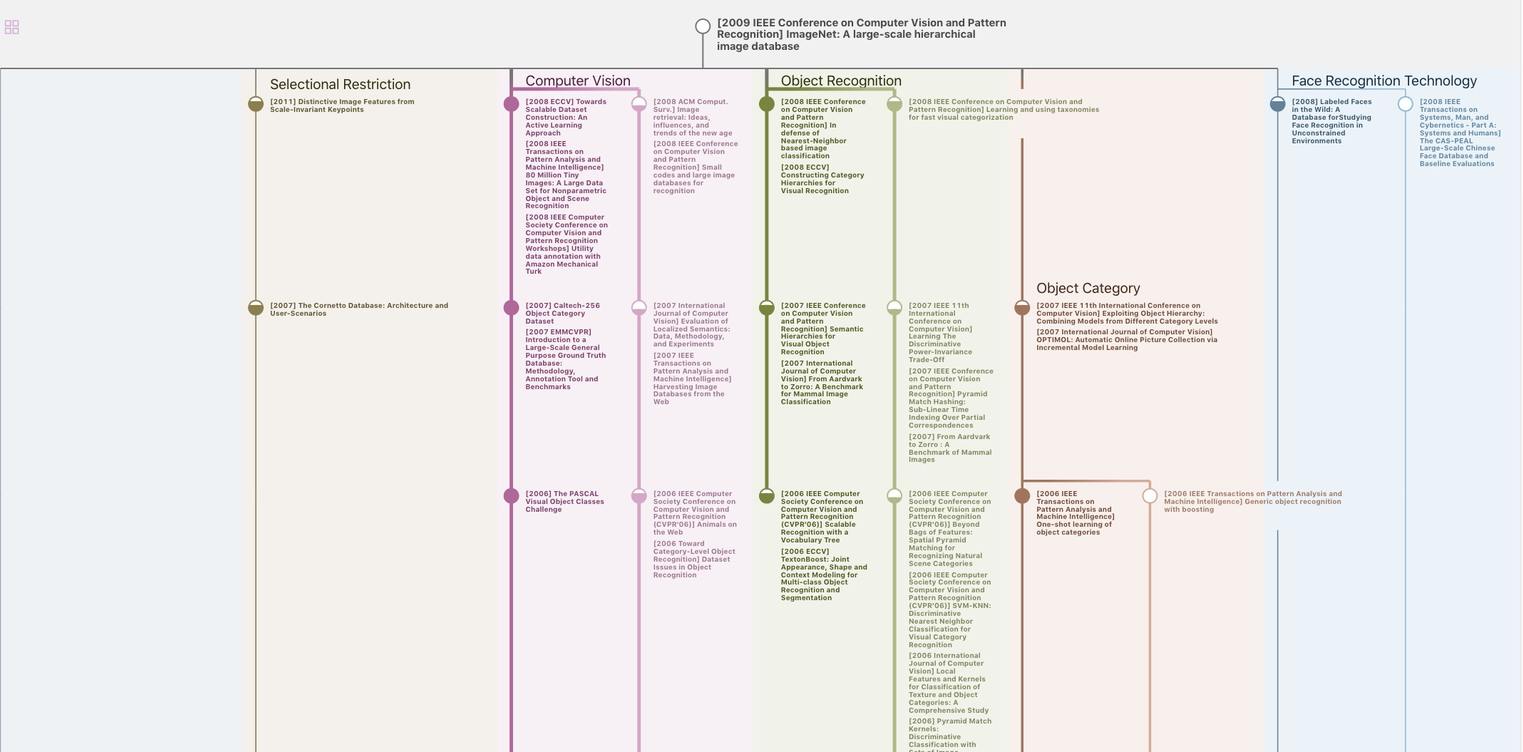
生成溯源树,研究论文发展脉络
Chat Paper
正在生成论文摘要