Structured World Belief for Reinforcement Learning in POMDP
INTERNATIONAL CONFERENCE ON MACHINE LEARNING, VOL 139(2021)
摘要
Object-centric world models provide structured representation of the scene and can be an important backbone in reinforcement learning and planning. However, existing approaches suffer in partially-observable environments due to the lack of belief states. In this paper, we propose Structured World Belief, a model for learning and inference of object-centric belief states. Inferred by Sequential Monte Carlo (SMC), our belief states provide multiple object-centric scene hypotheses. To synergize the benefits of SMC particles with object representations, we also propose a new object-centric dynamics model that considers the inductive bias of object permanence. This enables tracking of object states even when they are invisible for a long time. To further facilitate object tracking in this regime, we allow our model to attend flexibly to any spatial location in the image which was restricted in previous models. In experiments, we show that object-centric belief provides a more accurate and robust performance for filtering and generation. Furthermore, we show the efficacy of structured world belief in improving the performance of reinforcement learning, planning and supervised reasoning.
更多查看译文
关键词
pomdp,reinforcement learning,structured,world
AI 理解论文
溯源树
样例
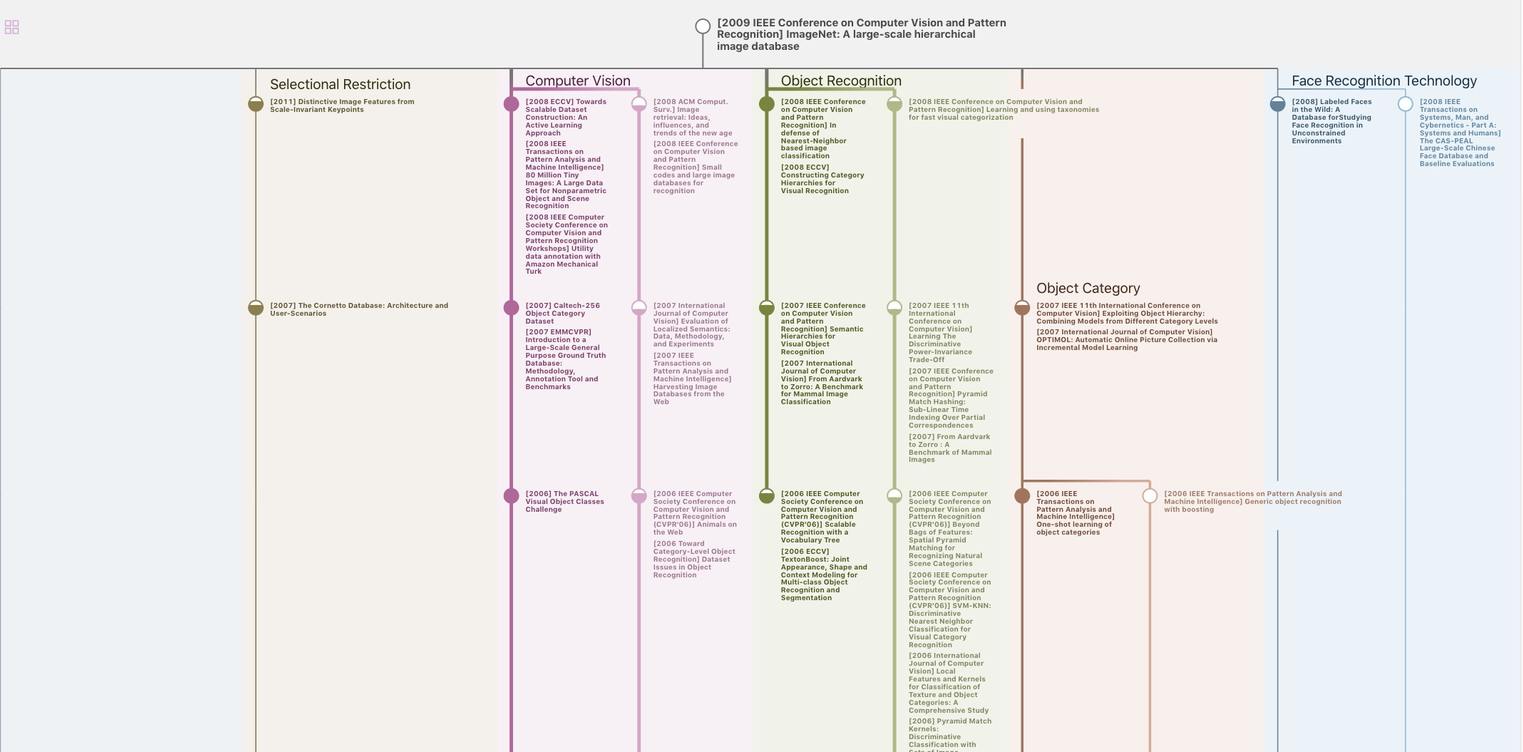
生成溯源树,研究论文发展脉络
Chat Paper
正在生成论文摘要