SPACE: Unsupervised Object-Oriented Scene Representation via Spatial Attention and Decomposition
ICLR(2020)
摘要
The ability to decompose complex multi-object scenes into meaningful abstractions like objects is fundamental to achieve higher-level cognition. Previous approaches for unsupervised object-oriented scene representation learning are either based on spatial-attention or scene-mixture approaches and limited in scalability which is a main obstacle towards modeling real-world scenes. In this paper, we propose a generative latent variable model, called SPACE, that provides a unified probabilistic modeling framework that combines the best of spatial-attention and scene-mixture approaches. SPACE can explicitly provide factorized object representations for foreground objects while also decomposing background segments of complex morphology. Previous models are good at either of these, but not both. SPACE also resolves the scalability problems of previous methods by incorporating parallel spatial-attention and thus is applicable to scenes with a large number of objects without performance degradations. We show through experiments on Atari and 3D-Rooms that SPACE achieves the above properties consistently in comparison to SPAIR, IODINE, and GENESIS. Results of our experiments can be found on our project website: https://sites.google.com/view/space-project-page
更多查看译文
关键词
Generative models, Unsupervised scene representation, Object-oriented representation, spatial attention
AI 理解论文
溯源树
样例
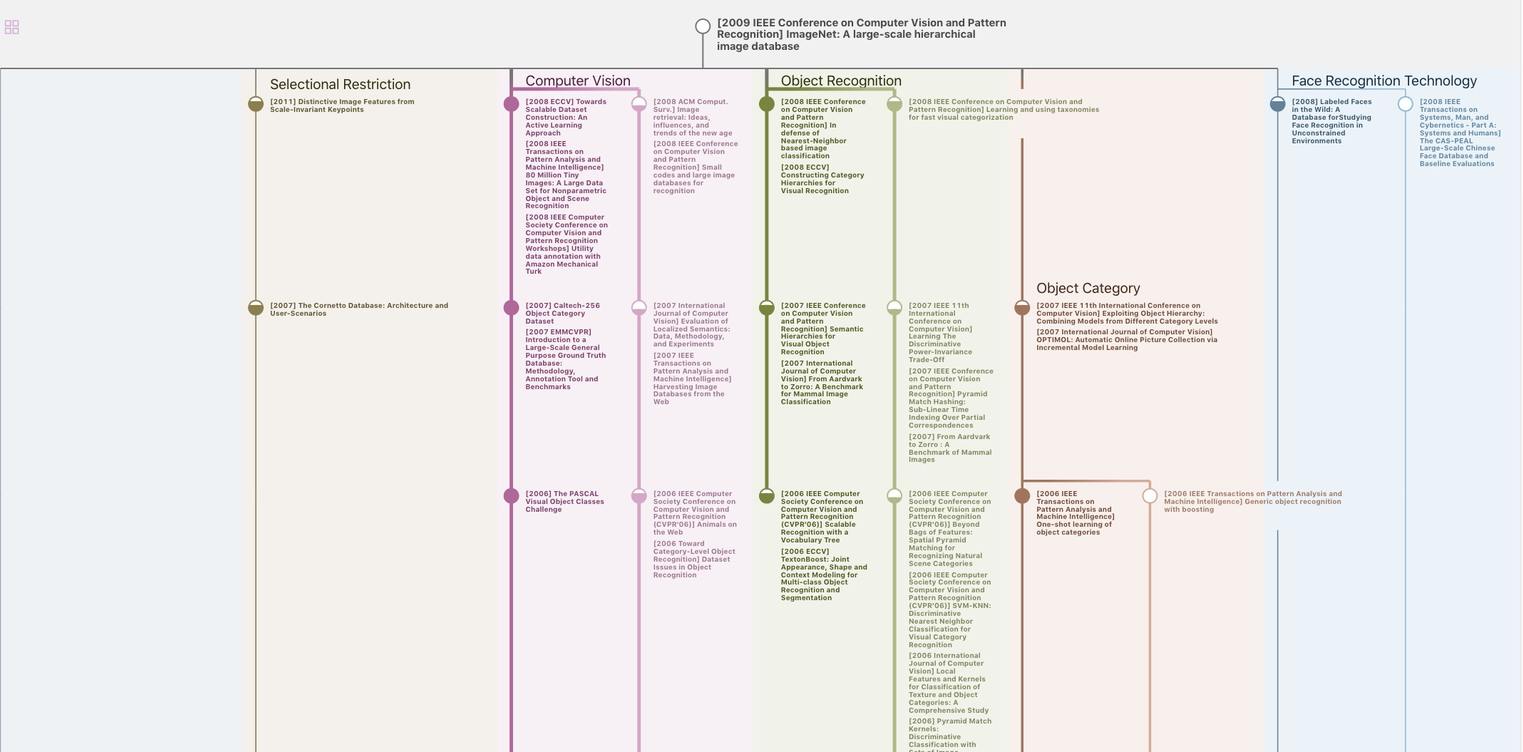
生成溯源树,研究论文发展脉络
Chat Paper
正在生成论文摘要