Scalable, Efficient Anonymization with INCOGNITO - Framework & Algorithm
2017 IEEE International Congress on Big Data (BigData Congress)(2017)
摘要
With the advent of "big-data" processing and analytics, organizations and enterprises have increased the collection of data from individuals, and are increasingly developing business models involving analytics to gain deep insights into the collected data. Often, it becomes essential to release and merge said data to third-parties for more extensive analytics for which an organization may not have the necessary expertise. Data often has to be anonymized prior to such release, to safeguard the privacy of individuals involved. While several algorithms, with varying privacy guarantees, have been proposed for anonymizing data, large scale distributed anonymization remains an under-explored topic. In this paper, we propose Incognito, a distributed algorithm and framework for anonymization of large data sets. Incognito as a framework is targeted at data center environments, both private data centers and public clouds, and is intended to be compatible with modern data analytics frameworks like mapreduce and resilient distributed datasets (RDDs). Incognito the algorithm aims at minimizing identity, similarity and skins based attacks on anonymized data sets. This paper describes Incognito in detail along with an empirical evaluation of its scalability and efficiency.
更多查看译文
关键词
anonymization,data privacy,big data,cloud computing
AI 理解论文
溯源树
样例
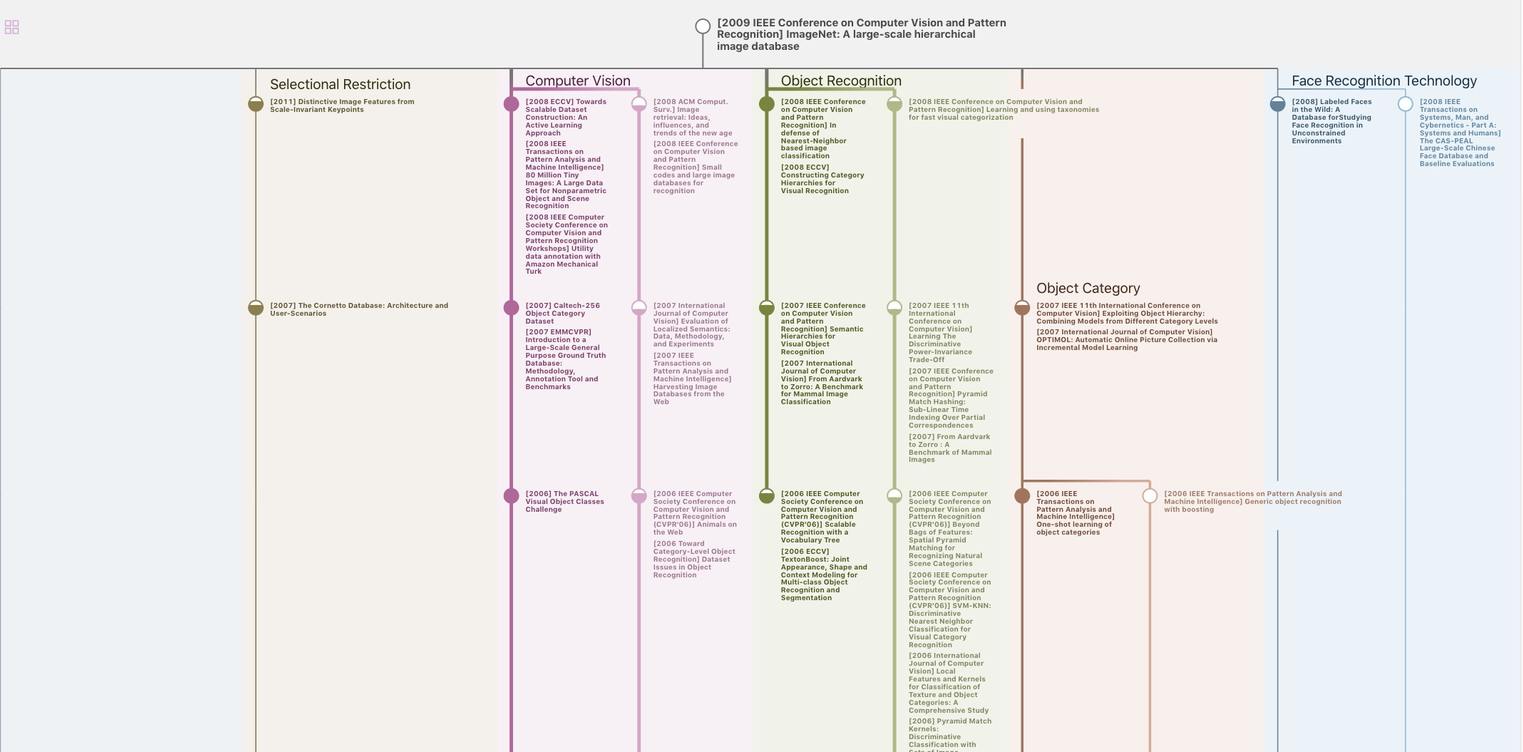
生成溯源树,研究论文发展脉络
Chat Paper
正在生成论文摘要